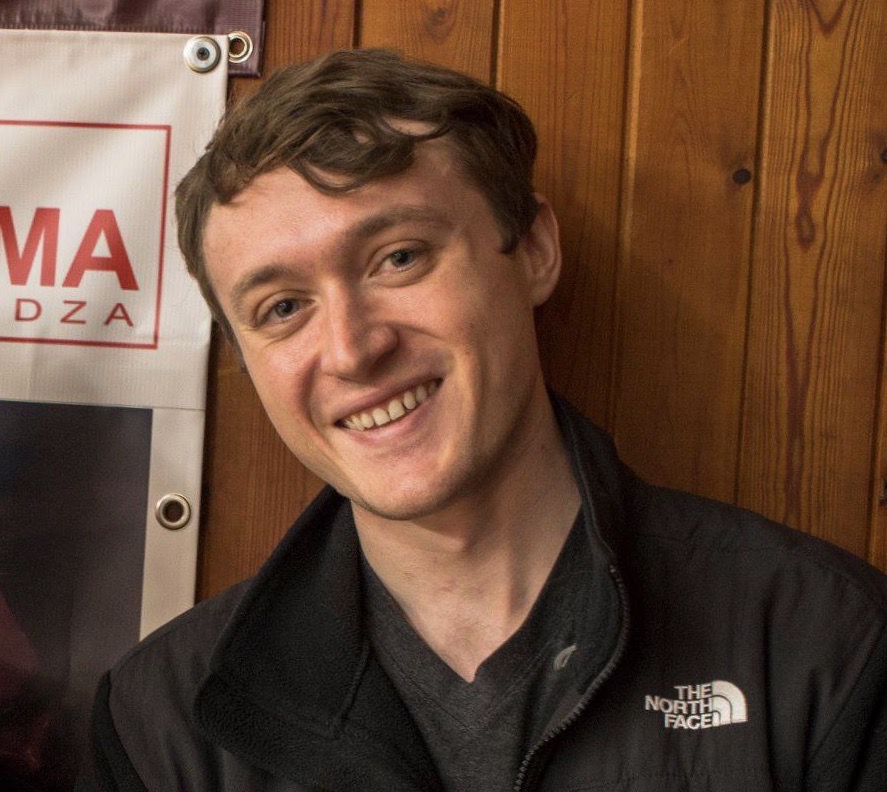
Education
AS, Natural Sciences and Mathematics, Bergen Community College, 2014
AS, Natural Sciences and Mathematics, Bergen Community College, 2014
BA, Biomathematics,Rutgers University, 2017
Ph.D. Student, Quantitative Biomedicine, Rutgers University, 2018 – present
Research Interests
Computational Protein Design, Molecular Evolution and Dynamics, Biophysics, Numerical Analysis
Computational Protein Design, Molecular Evolution and Dynamics, Biophysics, Numerical Analysis
Research Summary
Phylogenic trees provide researchers with a way to determine the evolutionary relationships between organisms. As a consequence, phylogenetic trees infer that all organisms on earth are connected to a single ancestor or, more specifically, a last common ancestor (LCA). Isolating the last known common ancestor is a difficulty in and of itself. For example, in the case of tropomyosin, a protein located in the cytoskeleton and muscle thin filaments in diverse metazoan phyla, the LCA is hypothesized to be ur-bilateria. However, in the case of certain metalloproteins and oxidoreductases, the answer is obscured by lateral gene events and selective pressures. In general, determining ancestry along this path becomes problematic due to the fact that phylogenetic trees and, thus, ancestry itself, relies on distances obtained from pairwise comparisons of sequence data. These pairwise sequence comparisons are used to generate a distance matrix, which is then utilized by various algorithms to construct phylogenic trees. Many of these algorithms may generate varying results for a similar set of data because of certain assumptions made during the algorithms construction. For example, a standard algorithm used to build phylogenic trees is called the unweighted pair group method using arithmetic mean (UPGMA). This particular method generates rooted trees, which assumes a constant molecular clock. Such an assumption is unrealistic, as the mutation rate of one organism's DNA is different from that of other organisms (e.g., humans and rats). Sequences diverge many times throughout the course of evolution, and molecular clocks become even more unreliable for increasing time scales because many DNA sites have undergone multiple site mutations. Therefore, not only is it hard enough as it is to align those particular sequences, but obtaining distances and building trees is also harder, and maybe even undoable altogether. One way to potentially circumvent this problem would be to consider a structure distance, such as the root mean square deviation (RMSD), instead of pairwise sequence distances. However, this is also unreliable as the RMSD is inversely proportional to the distances obtained by comparing pairwise sequence differences. Considering that structure is equivalent to function, would it then be possible to consider dynamics, and thus function, as another metric for attaining deeper time connections when characterizing evolutionary lineage? To explore this idea further, we have considered replicating previous experiments that have simulated the contractile tropomyosins of Penaeus monodons and Drosophila melanogaster over a 500-nanosecond trajectory. Once the simulation is completed, we will then go about calculating the subspace overlap between the two trajectories. The subspace overlap is but one of the measures used to compare the subspaces determined by both the Elastic Network Model (ENM) and Principal Component Analysis (PCA). The subspace overlap would be the first metric used in the construction of a dynamic phylogeny because it shows us ranges from 0, when the eigenvector subsets are completely dissimilar, to 1 (or 100%), when the two trajectories are completely identical. Thus, in proceeding, we seek to attain better time connections by incorporating dynamics, or subspace overlap, as an added metric. A majority of my work thus far has been centered around tropomyosin, but I intend to move away from tropomyosin so that I could begin working with ferredoxins. My lab is currently engineering ferredoxins as electron carriers in cells, and I would like to understand the evolution of this protein by utilizing the methods illustrated above so that I could figure out how to engineer better physical properties into it.
Phylogenic trees provide researchers with a way to determine the evolutionary relationships between organisms. As a consequence, phylogenetic trees infer that all organisms on earth are connected to a single ancestor or, more specifically, a last common ancestor (LCA). Isolating the last known common ancestor is a difficulty in and of itself. For example, in the case of tropomyosin, a protein located in the cytoskeleton and muscle thin filaments in diverse metazoan phyla, the LCA is hypothesized to be ur-bilateria. However, in the case of certain metalloproteins and oxidoreductases, the answer is obscured by lateral gene events and selective pressures. In general, determining ancestry along this path becomes problematic due to the fact that phylogenetic trees and, thus, ancestry itself, relies on distances obtained from pairwise comparisons of sequence data. These pairwise sequence comparisons are used to generate a distance matrix, which is then utilized by various algorithms to construct phylogenic trees. Many of these algorithms may generate varying results for a similar set of data because of certain assumptions made during the algorithms construction. For example, a standard algorithm used to build phylogenic trees is called the unweighted pair group method using arithmetic mean (UPGMA). This particular method generates rooted trees, which assumes a constant molecular clock. Such an assumption is unrealistic, as the mutation rate of one organism's DNA is different from that of other organisms (e.g., humans and rats). Sequences diverge many times throughout the course of evolution, and molecular clocks become even more unreliable for increasing time scales because many DNA sites have undergone multiple site mutations. Therefore, not only is it hard enough as it is to align those particular sequences, but obtaining distances and building trees is also harder, and maybe even undoable altogether. One way to potentially circumvent this problem would be to consider a structure distance, such as the root mean square deviation (RMSD), instead of pairwise sequence distances. However, this is also unreliable as the RMSD is inversely proportional to the distances obtained by comparing pairwise sequence differences. Considering that structure is equivalent to function, would it then be possible to consider dynamics, and thus function, as another metric for attaining deeper time connections when characterizing evolutionary lineage? To explore this idea further, we have considered replicating previous experiments that have simulated the contractile tropomyosins of Penaeus monodons and Drosophila melanogaster over a 500-nanosecond trajectory. Once the simulation is completed, we will then go about calculating the subspace overlap between the two trajectories. The subspace overlap is but one of the measures used to compare the subspaces determined by both the Elastic Network Model (ENM) and Principal Component Analysis (PCA). The subspace overlap would be the first metric used in the construction of a dynamic phylogeny because it shows us ranges from 0, when the eigenvector subsets are completely dissimilar, to 1 (or 100%), when the two trajectories are completely identical. Thus, in proceeding, we seek to attain better time connections by incorporating dynamics, or subspace overlap, as an added metric. A majority of my work thus far has been centered around tropomyosin, but I intend to move away from tropomyosin so that I could begin working with ferredoxins. My lab is currently engineering ferredoxins as electron carriers in cells, and I would like to understand the evolution of this protein by utilizing the methods illustrated above so that I could figure out how to engineer better physical properties into it.
Awards & Honors
Rutgers NIH Biotechnology Training Program Fellowship, Rutgers-New Brunswick 2019-2020
Rutgers NIH Biotechnology Training Program Fellowship, Rutgers-New Brunswick 2019-2020
Rutgers Presidential Fellowship, Rutgers - New Brunswick 2018-2019
Fulbright Award, Institute for International Education (IIE) 2018-2019
SAS Paul Robeson Scholar, Rutgers - New Brunswick Honors Program 2017
Gamma Sigma Alpha, Rutgers - New Brunswick 2015
Beacon Scholar, Bergen Community College 2014
Psi Beta, Bergen Community College 2013
Phi Theta Kappa (PTK), Bergen Community College 2012
Publications
Lindsay, RJ, Siess J, Lohry DP, McGee TS, Ritchie JS, Johnson QR, Shen T. Characterizing protein conformations by correlation analysis of coarse-grained contact matrices. J Chem Phys. 2018;148(2):025101. doi: 10.1063/1.5004141. PMID: 29331124
Lindsay, RJ, Siess J, Lohry DP, McGee TS, Ritchie JS, Johnson QR, Shen T. Characterizing protein conformations by correlation analysis of coarse-grained contact matrices. J Chem Phys. 2018;148(2):025101. doi: 10.1063/1.5004141. PMID: 29331124
Representative Graduate Courses Taken
Special Topics in Physical Chemistry: Thermodynamics and Kinetics
Special Topics in Physical Chemistry: Thermodynamics and Kinetics
Pharmaceutical Materials Engineering
Bioinformatics