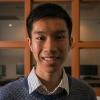
Education
BS, Computer Science and Engineering, University of California, Los Angeles, 2019
PhD Candidate, Quantitative Biomedicine, Rutgers University, 2020 - Present
Research Interests
Single cell analysis, cancer, clonal evolution, statistical modeling, machine learning, high-throughput sequencing, bioinformatics
Research Summary
Cancer follows the process of clonal expansion, where accumulation of mutations in subpopulations of cells in both a branched and linear manner results in heterogeneous tumors. This genetic heterogeneity contributes to highly complex interactions that affect prognosis of the disease and predict responses to therapy. Recently, it has been shown that similar mutational processes are also active in non-tumor populations. Moreover, its detection prior to chemotherapy has suggested a permissive role in therapy-related secondary myeloid disease development in cancer patients. High-throughput sequencing technologies and single-cell analysis have been used to explore these complex clonal dynamics within the tumor environment and demonstrate that the presence of clonal evolution correlates with poor prognosis and progression of primary malignancy. My research focuses on evaluating cellular heterogeneity in cancer through analyzing single-cell transcriptional profiles and elucidating its interactions with cancer and subsequent therapy.
Single cell sequencing data is subject to noise defined as highly stochastic levels of low and zero expression gene counts, which is caused by both biological factors and experimental conditions such as inconsistent RNA capture rate. This noise and the resulting sparsity of the data complicates data analysis and obscures biological signals. Several computational techniques and algorithms, based on both statistical modeling and machine learning, have been designed to remedy noise in single-cell data and improve analyses such as cell type identification, differential gene expression analysis, and trajectory inference to varying degrees of success. As a result, in addition to utilizing single cell analysis to explore clonal evolution and cellular heterogeneity in cancer, I also aim to explore the quantitative methods involved as part of my research. I wish to both evaluate their underlying statistical principles as well as understand how to interpret them in a biologically meaningful way, as the choice of quantitative methods and their parameters greatly affects the biological conclusions made from further data analysis.